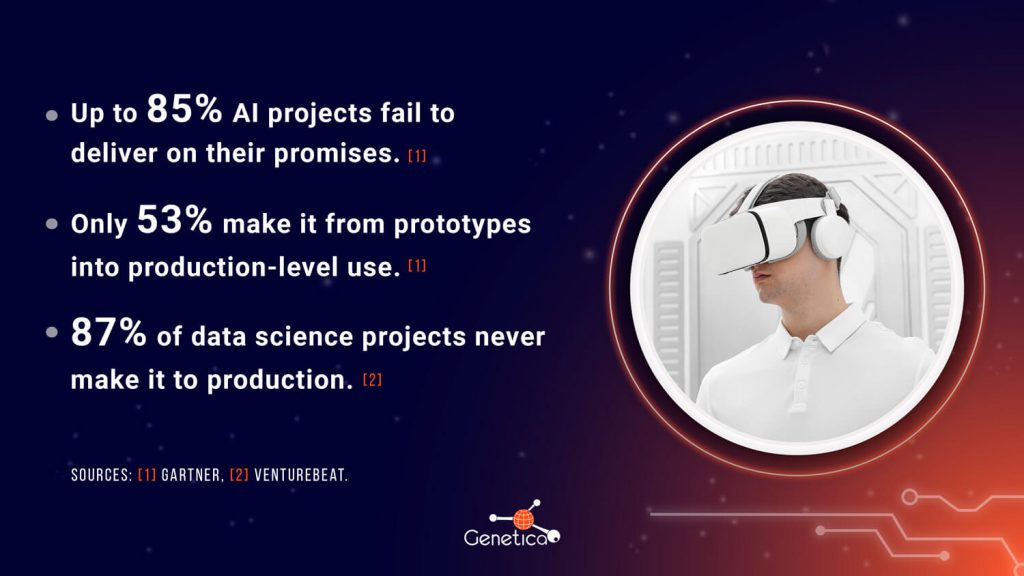
There has been significant excitement around artificial intelligence (AI) – and for good reasons. When done well, AI can provide businesses with essential insights and help them automate processes to increase efficiency and gain a competitive advantage. However, Gartner’s research has revealed that up to 85% fail to deliver on their promises, while only 53% make it from prototypes into production-level use! Further, according to VentureBeat (2019), 87% of data science projects never make it to production. Here are some valuable insights from industry experts on how not to become a failed statistic and maximize ROI.
Here’s why AI projects fail
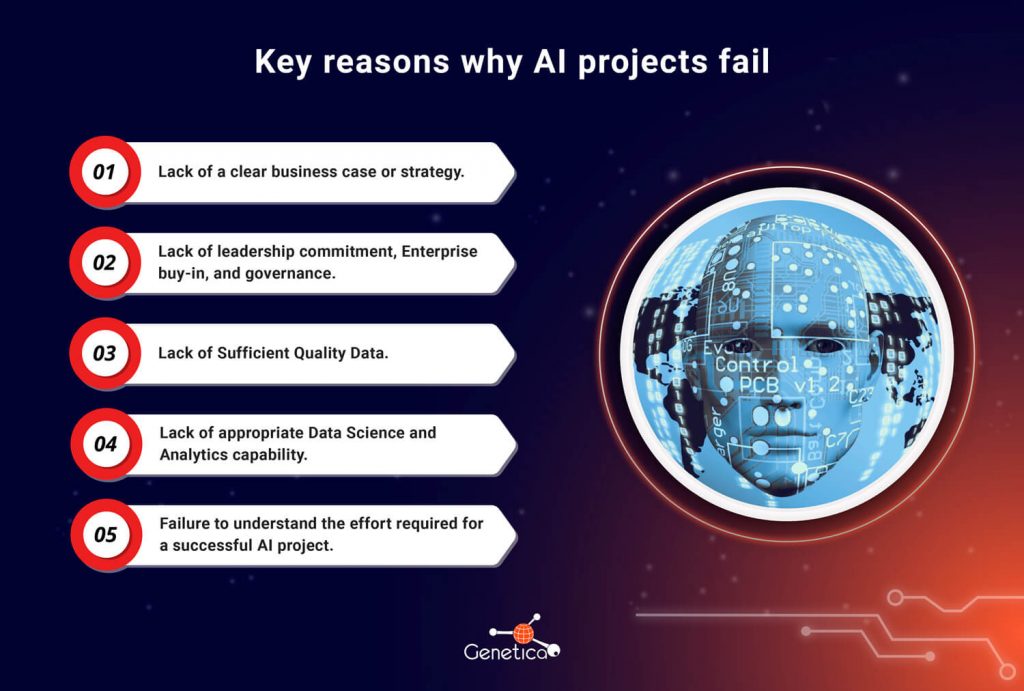
Lack of a clear business case or strategy
A recent study by MIT Sloan revealed that most AI projects fail due to a lack of a clear business case or strategy. This is not surprising, given the fact that AI is still a relatively new technology, and many organizations are still trying to figure out how to utilize it best. However, the study also found that organizations with a clear business case for their AI projects were significantly more likely to succeed.
For example, if an organization wants to use AI to improve customer service, it must first define what that means and what metrics it’ll use to measure success. Without a clear goal, it’s easy for an AI project to lose its way and become bogged down in technical details.
Lack of leadership commitment, Enterprise buy-in, and governance
To bring an AI project to fruition, it is essential to have strong leadership support and a well-defined governance structure. Unfortunately, many organizations lack both of these critical components. For an AI project to be successful, In addition to strong leadership commitment, it’s crucial to have enterprise buy-in from the start. Furthermore, a practical governance framework must be in place to ensure the project stays on track.
Lack of Sufficient Quality Data
AI is only as good as the data it relies on. If that data is insufficient or of poor quality, the AI will also be. This is one of the primary reasons why so many AI projects fail to meet expectations.
- Poor quality data can lead to inaccurate results and incorrect decisions.
- The AI will make inaccurate predictions if the data is not representative of the overall population.
- Insufficient data can hamper an AI’s ability to learn and evolve. As a result, AI may become less effective over time.
Lack of access to appropriate Data Science and Analytics skills
There is still a significant lack of access to appropriate Data Science and Analytic skills. In the absence of having access to the traditional number of resources required for an AI project, organizations who attempt to implement AI experiences significant cost overruns and time delays (even if they were able to complete the project. Without access to these costly and scarce resources, organizations need to leverage platforms that eliminate this high dependency.
Failure to understand the effort required for a successful AI project
One of the main reasons why AI projects fail is because people have unrealistic expectations about what AI can do. People often believe that AI is a magic bullet that can solve all their problems. However, AI is not a panacea, and it has its limitations. It’s important to understand those limitations to set realistic expectations for an AI project.
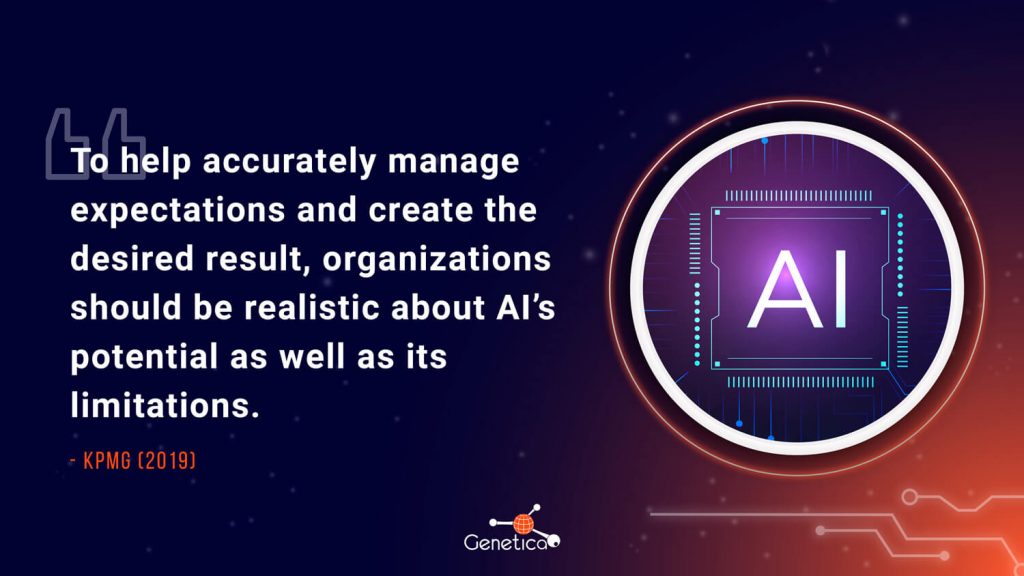
“To help accurately manage expectations and create the desired result, organizations should be realistic about AI’s potential as well as its limitations.”
KPMG (2019)
How Genetica is helping businesses to avoid these common pitfalls
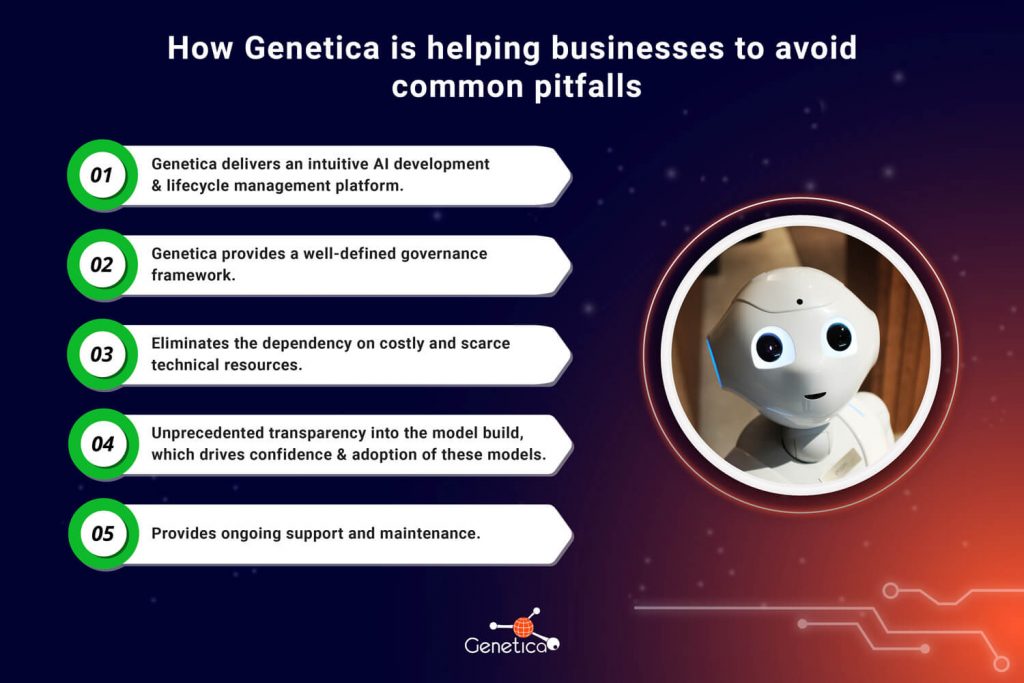
Genetica’s Cortex Cognitive AI Platform is a cloud-based end-to-end AI development & lifecycle management solution. Our intuitive UI & zero programming environment is ideal for developing, deploying, and maintaining industrial-strength AI applications.
Once a model is deployed Genetica not only manages ‘what’ needs to be done it also recommends ‘how’ and by ‘whom’ autonomously.
Let Genetica help you take your business to the next level with AI.